Day 2 : Open AI’s Reinforcement Fine-Tuning
Day 2/12 OpenAI: Reinforcement Fine-Tuning
OpenAI’s 12 series has recently announced its Day 2 of the Research Program — Reinforcement Fine-Tuning (RFT) which marks a pivotal moment in AI development, particularly for its O1 series of models. This innovative approach promises to enhance the customization of AI systems, allowing organizations to create highly specialized models with minimal training data. As AI becomes increasingly integrated into various sectors, understanding the implications of RFT is crucial for developers, researchers, and businesses alike.
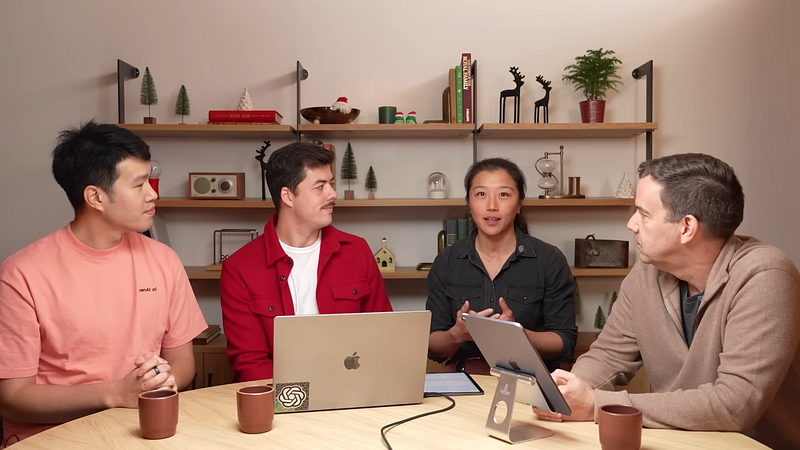
What Is OpenAI’s Reinforcement Fine-Tuning?
Reinforcement Fine-Tuning is a method that allows developers to adapt OpenAI’s models for specific tasks by utilizing a reward-driven training loop. Unlike traditional fine-tuning methods that require extensive datasets, RFT enables the creation of effective models using only a few examples. This capability is particularly beneficial for complex applications in fields such as law and healthcare.
Learning with Few Examples
One of the standout features of RFT is its ability to learn from minimal examples. For instance, during a demonstration by Berkeley Lab, a model was trained to diagnose rare genetic diseases using just dozens of clinical cases. This efficiency challenges the conventional requirement of thousands of examples typically needed for training, showcasing how RFT can significantly reduce the time and resources required for model development.
How OpenAI’s New Approach Changes Everything?
OpenAI’s RFT represents a strategic shift in AI development. Traditionally, the focus has been on building increasingly powerful foundational models. However, RFT redistributes the power to create specialized AI systems across various industries. This new approach not only enhances technical capabilities but also transforms how organizations implement and optimize AI solutions for specific domains.
Why It Matters?
The significance of RFT lies in its potential to democratize access to advanced AI capabilities. By enabling organizations to tailor models to their unique needs without extensive training data, RFT can accelerate innovation across sectors. This shift could lead to more efficient problem-solving in fields like medicine, law, and education.
How Does It Work?
RFT operates by rewarding models for following expert reasoning processes rather than merely memorizing patterns. The training involves presenting the model with specific tasks and providing feedback based on its performance. This iterative process helps refine the model’s ability to generate accurate responses based on limited input.
Who Benefits?
The beneficiaries of OpenAI’s RFT include:
- Researchers: Can develop specialized models for niche applications.
- Businesses: Gain the ability to create tailored solutions that enhance operational efficiency.
- Developers: Have access to tools that simplify the customization of AI systems without extensive resources.
OpenAI’s Approach Reveals About Expertise Itself
OpenAI’s reinforcement fine-tuning provides insights into the nature of expertise. By teaching machines how experts think rather than just what they know, this approach illuminates the decision-making processes that underpin expert knowledge. This revelation could influence not only AI development but also educational methodologies aimed at fostering expertise in humans.
Using OpenAI’s Reinforcement Fine-Tuning to Customize ChatGPT o1 (mini)
To utilize RFT for customizing ChatGPT o1 (mini), users need:
- Training Data: A small dataset relevant to the specific application.
- Validation Data: To test for overfitting and ensure model robustness.
- Grader Configuration: To define evaluation metrics that guide the reinforcement process.
This setup allows developers to efficiently adapt ChatGPT for various tasks while maintaining high performance with limited data.
Challenges to Consider
Despite its advantages, RFT comes with challenges:
- Stability: Reinforcement learning can be unpredictable, requiring careful management during training.
- Understanding Best Practices: Developers may need time to familiarize themselves with effective strategies for implementing RFT.
- Resource Allocation: While less data is needed, adequate resources must still be allocated for model training and testing.
Future of Reinforcement Fine-Tuning
The future of reinforcement fine-tuning looks promising as it continues to evolve. With ongoing improvements in stability and usability, we can expect broader adoption across various fields. As organizations become more adept at leveraging this technology, it may redefine how we approach AI specialization and application in real-world scenarios.
Conclusion
OpenAI’s Reinforcement Fine-Tuning heralds a transformative era in AI development. By enabling more efficient learning from fewer examples and promoting expert-like reasoning in machines, this approach not only enhances technical capabilities but also democratizes access to advanced AI tools. As we stand on the brink of this new frontier, embracing these innovations will be crucial for those looking to harness the full potential of AI in their respective fields.
Ready to build your tech dream team?
Check out MyNextDeveloper, a platform where you can find the top 3% of software engineers who are deeply passionate about innovation. Our on-demand, dedicated, and thorough software talent solutions are available to offer you a complete solution for all your software requirements.
Visit our website to explore how we can assist you in assembling your perfect team.